Modern businesses face complex challenges that require informed, forward-looking decisions. Predictive analytics has become a critical tool for companies seeking to make better strategic choices.
At its core, predictive analytics uses historical data, statistical algorithms, and machine learning techniques to identify the likelihood of future outcomes. This approach transforms raw data into valuable insights that guide business strategy.
This article explores how organisations can effectively implement predictive analytics to enhance their decision-making processes. We’ll examine how companies like Kanda Software apply these principles across different business functions and discuss implementation strategies.
What Is Predictive Analytics?
Predictive analytics represents the intersection of statistics, machine learning, and business intelligence. At its fundamental level, it uses patterns found in historical data to identify risks and opportunities for the future. Unlike traditional business intelligence that answers “what happened,” predictive analytics addresses “what will likely happen” and, when combined with prescriptive elements, “what should we do about it.”
The field has evolved significantly over the past decades. What began as simple statistical forecasting has transformed into sophisticated machine learning approaches that can process vast amounts of unstructured data and identify complex patterns humans might miss. This evolution parallels advances in computing power, data storage capabilities, and algorithm development.
The Predictive Analytics Process
A successful predictive analytics implementation typically follows this workflow:
Business understanding: Defining clear objectives and success metrics. Data collection: Gathering relevant information from internal and external sources. Data preparation: Cleaning, transforming, and organising raw data. Exploratory analysis: Investigating relationships and patterns in the data. Feature engineering: Creating variables that best represent the underlying patterns. Model selection and training: Choosing and building appropriate algorithms. Model evaluation: Testing performance against validation data. Deployment: Integrating the model into business systems. Monitoring and refinement: Tracking performance and updating as needed.Each stage requires collaboration between data scientists, IT specialists, and business stakeholders to ensure technical soundness and business relevance.
Types of Predictive Models
Several types of predictive models serve different business needs:
Regression models: These predict numerical values and answer questions like “How much will this customer spend?” or “What will next quarter’s sales be?” Linear regression serves as the foundation, while more complex variants like polynomial and multivariate regression handle non-linear relationships and multiple variables. Classification models: When you need to predict categories rather than numbers, classification models excel. They can determine whether an email is spam, if a transaction is fraudulent, or which product category a customer will purchase next. Popular techniques include logistic regression, decision trees, random forests, and support vector machines. Time series models: These specialised models account for temporal dependencies in data. ARIMA (AutoRegressive Integrated Moving Average), SARIMA (Seasonal ARIMA), and Prophet models help forecast metrics like website traffic, energy consumption, or inventory needs that follow time-based patterns. Clustering models: While technically unsupervised learning, clustering often supports predictive efforts by grouping similar entities. K-means, hierarchical clustering, and DBSCAN algorithms help segment customers, identify product categories, or find anomalies that might indicate fraud. Deep learning models: Neural networks with multiple layers can detect complex patterns in large datasets. Convolutional neural networks (CNNs) excel at image analysis, while recurrent neural networks (RNNs) and transformers handle sequential data like text and time series. Ensemble methods: These combine multiple models to improve prediction accuracy and robustness. Techniques like bagging (random forests), boosting (XGBoost, LightGBM), and stacking blend predictions from different algorithms to achieve superior performance.Each model type has specific use cases and strengths. The right choice depends on your business question, available data, and desired outcome.
Data Requirements
For predictive analytics to work effectively, you need:
Quality data: Accurate, complete, relevant, and consistent. Sufficient volume: Enough examples to train reliable models. Variety: Different data types and sources for comprehensive insights. Historical records: Past data that shows patterns over time. Relevant features: Variables that actually influence the outcome you want to predict.Without solid data foundations, even the most sophisticated algorithms will produce unreliable results. As the saying goes, garbage in, garbage out.
Business Applications of Predictive Analytics
Sales and Marketing Optimisation
Predictive analytics transforms sales and marketing by enabling:
Customer segmentation: Group customers based on behaviour, preferences, and value. Lead scoring: Identify prospects most likely to convert. Churn prediction: Flag customers at risk of leaving. Personalised recommendations: Suggest relevant products based on past behaviour. Marketing campaign optimisation: Determine which channels and messages will be most effective. Pricing optimisation: Set prices that maximise revenue and profit.For example, an e-commerce company might use purchase history and browsing behaviour to predict which products a customer is likely to buy next. These insights allow for targeted promotions that significantly improve conversion rates.
Financial Forecasting and Risk Management
In finance, predictive analytics helps with:
Cash flow forecasting: Project future financial needs. Credit scoring: Assess the likelihood of default or late payment. Fraud detection: Identify suspicious transactions in real-time. Investment analysis: Predict returns and optimise portfolios. Budget planning: Create more accurate financial projections.Financial institutions use machine learning algorithms to detect fraudulent credit card transactions by analysing patterns that humans might miss. These systems can evaluate thousands of transactions per second and flag suspicious activity instantly.
Supply Chain Management
Predictive analytics improves supply chain efficiency through:
Demand forecasting: Predict future product demand Inventory optimisation: Maintain optimal stock levels Supplier performance prediction: Identify potential issues before they occur Logistics optimisation: Plan the most efficient delivery routes Maintenance scheduling: Predict when equipment will need servicingA retail chain might analyse historical sales data, seasonal trends, and external factors like weather and local events to forecast demand for specific products. This helps them stock the right amounts in the right locations, reducing both stockouts and excess inventory.
Human Resources and Workforce Planning
HR departments benefit from predictive analytics by:
Talent acquisition: Identify the best candidates for open positions. Employee retention: Predict which employees might leave. Performance prediction: Forecast which employees will succeed in specific roles. Workforce planning: Project future staffing needs. Training effectiveness: Measure and predict the impact of learning programs.Companies like IBM use predictive models to identify employees at risk of leaving. By analysing factors such as commute distance, job role, compensation, and performance reviews, they can take proactive steps to retain valuable talent.
Operational Efficiency
Across operations, predictive analytics drives efficiency through:
Process optimisation: Identifying bottlenecks and improvement opportunities. Equipment maintenance: Predicting failures before they happen. Quality control: Forecasting potential defects. Resource allocation: Optimising how people and assets are deployed. Energy management: Predicting usage patterns to reduce costs.Manufacturing plants use sensors and predictive models to implement predictive maintenance, fixing equipment before it breaks down. This approach can reduce maintenance costs and decrease equipment downtime.
Implementation Strategy
Defining Clear Business Objectives
Successful predictive analytics starts with clear business goals. Ask:
What specific business problem are we trying to solve? What decisions will be improved with better predictions? How will we measure success? What is the potential business value of better predictions?For example, instead of vaguely wanting to “use AI for marketing,” a specific objective might be “reduce customer acquisition costs by 15% by identifying the prospects most likely to convert.”
Building the Right Team
Effective predictive analytics requires a mix of skills:
Data scientists: For building and validating models. Data engineers: For data pipeline development and management. Domain experts: For business context and interpreting results. IT specialists: For infrastructure and integration. Project managers: For coordinating efforts and tracking progress. Executive sponsors: For providing resources and organisational support.Depending on your organisation’s size and resources, you might build an internal team, work with external partners, or use a hybrid approach.
Choosing the Right Technology Stack
Your technology needs will depend on your specific goals, but often include:
Data storage solutions: Data warehouses, data lakes, or specialised databases. Data processing tools: ETL (Extract, Transform, Load) tools or data pipelines. Analytics platforms: From simple statistical packages to comprehensive machine learning platforms. Visualisation tools: For communicating insights effectively. Deployment infrastructure: For operationalising models.Options range from open-source tools like Python, R, and TensorFlow to commercial platforms from vendors like Microsoft, Google, Amazon, IBM, and specialised analytics providers.
Data Collection and Preparation
Data preparation often consumes 60-80% of a predictive analytics project. Here are the main steps to follow:
Identify data sources: Internal systems, third-party data, and public datasets. Extract and consolidate data: Bring data together from various sources. Clean the data: Address missing values, outliers, and inconsistencies. Transform the data: Convert data into formats suitable for analysis. Create features: Develop variables that will help predict outcomes. Split data: Separate into training and testing sets.This phase requires patience and attention to detail, as the quality of your prepared data directly impacts model performance.
Model Development and Validation
Developing effective predictive models involves:
Selecting appropriate algorithms: Based on the problem type and data characteristics. Training models: Using historical data to teach the model patterns. Tuning parameters: Adjusting settings to optimise performance. Validating results: Testing the model on data it hasn’t seen before. Evaluating performance: Using appropriate metrics (accuracy, precision, recall, etc.). Comparing models: Testing different approaches to find the best performer.It’s important to balance model complexity with interpretability. A slightly less accurate model that business users can understand may be more valuable than a “black box” with marginally better performance.
Deployment and Integration
Making predictive analytics operational requires:
Integrating with existing systems: Connecting models to data sources and business applications. Automating the prediction process: Setting up regular runs or real-time predictions. Creating user interfaces: Developing dashboards or reports for business users. Establishing alerts and workflows: Triggering actions based on predictions. Documenting the system: Ensuring knowledge transfer and maintenance.The goal is to make predictions available at the right time, in the right format, to the people who need them for decision-making.
Monitoring and Continuous Improvement
Predictive analytics is not a “set and forget” solution. You’ll need to take more action:
Track model performance: Monitor accuracy and other relevant metrics. Watch for concept drift: Identify when models become less accurate over time. Retrain regularly: Update models with new data. Refine features: Add or modify variables to improve performance. Collect feedback: Learn from users about prediction quality and usefulness. Update processes: Continually refine how predictions are used in decision-making.Regular review cycles ensure your predictive capabilities stay relevant as business conditions change.
Challenges and Solutions
Challenge Solutions Data quality and access issues— poor data quality or difficulty accessing relevant data
Implement data governance practices Invest in data cleaning and preparation tools Develop data quality metrics and monitoring Start with smaller projects using available data while building a better data infrastructure Consider third-party data to supplement internal sources Organisational resistance— reluctance to adopt data-driven decision-making
Start with pilot projects that demonstrate clear value Focus on augmenting rather than replacing human judgment Provide training on how to interpret and use predictions Involve stakeholders early in the process Celebrate and publicise success stories Technical complexity— predictive analytics requires specialised skills and infrastructure
Consider starting with user-friendly commercial tools Leverage cloud-based solutions that reduce infrastructure needs Invest in training for existing staff Partner with universities or consultants for specialised expertise Build capabilities incrementally rather than attempting everything at once Ethical and privacy concerns — ensuring predictions are used ethically and comply with regulations Develop clear ethical guidelines for analytics use Implement privacy by design principles Regular review of models for bias or fairness issues Transparency with customers and stakeholders about data use Stay current with relevant regulations (GDPR, CCPA, etc.) Model interpretability — complex models can be difficult to explain and trust Use simpler models when transparency is critical Implement explainable AI techniques Create visualisations that help non-technical users understand predictions Develop confidence metrics to accompany predictions Document model limitations and assumptionsFuture Trends in Predictive Analytics
Automated Machine Learning (AutoML)
AutoML tools automate the process of selecting algorithms, tuning parameters, and evaluating models. This democratises predictive analytics, allowing business users without data science expertise to build effective models. Platforms like Google’s AutoML, DataRobot, and H2O.ai are making these capabilities accessible to more organisations.
Edge Analytics
As Internet of Things (IoT) devices proliferate, more predictive analytics will happen at the “edge” — directly on devices rather than in centralised data centres. This enables real-time predictions without constant connectivity and reduces data transfer volumes. Applications include autonomous vehicles, smart manufacturing equipment, and remote monitoring systems.
Explainable AI
As predictive models become more integrated into critical business processes, the need for transparency increases. Explainable AI techniques help users understand why a model made a specific prediction. This builds trust, helps identify potential biases, and makes it easier to improve models based on business feedback.
Augmented Analytics
Augmented analytics combines predictive capabilities with natural language processing and generation. This allows business users to interact with data using conversational language, automatically generates insights from data, and explains findings in plain language. Platforms like Tableau, Power BI, and Thoughtspot are incorporating these capabilities.
Prescriptive Analytics
The next frontier moves beyond prediction (what will happen) to prescription (what should be done). Prescriptive analytics not only forecasts outcomes but also recommends actions to optimise those outcomes. This often involves simulation and optimisation techniques that evaluate multiple scenarios and identify optimal decision paths.
Conclusion
Predictive analytics has moved from a competitive advantage to a business necessity. Organisations that effectively implement these capabilities can make faster, more informed decisions that drive better outcomes across all business functions.
The key to success lies not just in the technology but in how you apply it to specific business challenges. Start with clear objectives, invest in data quality, build the right team, choose appropriate tools, and focus on continuous improvement. Most importantly, ensure that predictive insights actually influence decision-making processes rather than becoming interesting but unused information.
Predictive technologies continue to advance, so organisations that build strong foundations now will be well-positioned to adopt emerging capabilities and maintain their competitive edge. The future belongs to companies that not only collect data but transform it into actionable insights that guide strategic direction.
The post Harnessing Predictive Analytics for Strategic Business Decision-Making appeared first on IoT Business News.
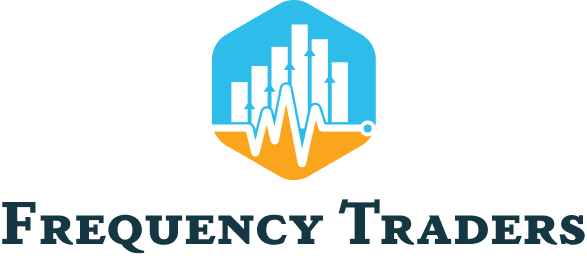